The weight of our beliefs: on the merits of a weighing scale as a metaphor for the Bayesian Brain
Note: This essay was written during my Master’s degree for the course Neurophilosophy and Ethics. I got a 7.5 (B+) and thought I would share it.
Human senses are incredibly limited and fallible. We only experience a small fraction of all the existing sounds and light frequencies (for instance, we see between 400 nm to 700 nm out of an infinite electromagnetic spectrum)1, but even more fundamentally than that, the perception of a ‘true reality’ is inherently impossible because all information that arrives into our consciousness is inexorably multivariate.2
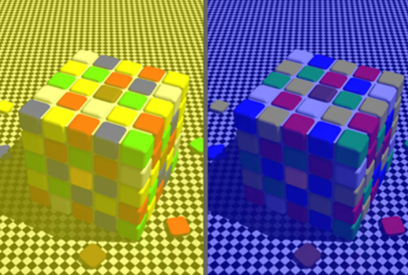
Figure 1. The yellow stickers on the left panel are the exact same shade of grey as the blue stickers on the right panel. Due to the inverse problem, our brain makes a probabilistic decision about what color to see based on previous experiences.
The fundamental ambiguity of our perception of reality, the so-called ‘inverse problem’ was first described in detail by Hermann von Helmhortz3, and it can be exemplified by our sense of vision: what we perceive as ‘color’ comprises three elements: 1) the light characteristics that arrive from a light source; 2) the way that they bounce off of an object and; 3) and the air between the object and our eyes. This means that what we perceive as color has three indistinguishable variables: illumination (which is intrinsic to the light source), reflectance (how the object interacts with light) and transmittance (the influence of the atmosphere between object and eye). As a consequence, our brains need to make judgments about ‘which color to see’, which can result in interesting optical illusions (Figure 1)2. Similar problems of multivariability exist for all of our senses, which means that reality is fundamentally inacessible to our brains.
This seems counterintuitive since humans are arguably the most successful species that have ever existed: we are on the top of our food chain, we lack natural predators and we are capable of inhabiting virtually every ecosystem on earth4 — which must entail that we have been able to adequately navigate our environment. How is it possible that we can appropriately change our behaviors based on our perception of reality if reality itself cannot be assessed by our senses? One possible answer is that we have a Bayesian brain — we make decisions on experiential information and construct our beliefs and decide courses of action based on the probability that they are correct.
Figure 2. A weighing scale can be used as a metaphor for the Bayesian brain.
A metaphor that could explain the principle behind the Bayesian brain is the following: Imagine an old school weighing scale, where objects can be put on each side to verify which one is the heaviest (Figure 2). This can serve as a visual metaphor for the way our brains make decisions based on probabilities: we attribute ‘weight’ to pieces of evidence that we encounter over our lifetime and whichever side of a belief has the most weight, ‘wins’ – becoming the thing that we believe.
One could explain this principle with an extremely simplified binary example: the belief “I like or I do not like bananas” are on the two ends of a scale. Our brain makes the decision about which one to believe (and then act upon) by weighing the evidence for “I like bananas” (maybe I have eaten hundreds of bananas and I always enjoyed them) and the opposing evidence for “I don’t like bananas” (maybe I once ate a banana with a worm in it) against each other. Each ‘pebble of evidence’ has its own weight and whichever side of the scale has the most weight, wins over the other side and becomes the belief we hold. Every new experience with bananas gives another ‘pebble of evidence’ in the scale — which means that we are constantly updating our beliefs based on new evidence, and then changing our behavior based on that updated scale.
Some researchers believe that the principle behind this analogy applies to how the entire brain works!5–8 Every sense that we have (smell, touch, vision) do not represent reality: they represent a set of beliefs that we have obtained through experience in the natural world, which we use as a way to make decisions. A simple example of the role of experience in our perception is the fact that humans tend to interpret nearly-vertical lines as completely vertical and nearly-horizontal lines as completely horizontal – because these are the patterns most commonly observed in nature.9
Figure 3. The Necker cube is an example of bistable perception.
This metaphor has many great features: as with a normal scale, it can be in equilibrium — e.g. for the belief ‘I like or I do not like bananas’, if your experiential evidence is equivalent for both sides, you may come to the conclusion ‘I don’t mind bananas, I do not like them but I do not dislike them either’. As applied to our sense of vision, one could imagine this state of ‘belief equilibrium’ exemplified by the Necker cube, in which we have a bistable perception about which face is closest to us (either the lower-left or the upper-right)10 – which could be analogous to the scale slightly wobbling from left to right depending on which part of the figure we choose to focus on.
Another good feature of the metaphor is that the distinction between the number of pieces of evidence and their ‘value’ makes intuitive sense: if you have 100 pebbles that weigh 1 g each and one pebble that weighs 1000 g, should you place them on opposite sides of the scale, the single heavier pebble will win. Similarly, 100 good experiences may be outweighed by one horrible experience in determining one’s belief about the fruit (for instance, eating a banana with a worm in it, in the decision ‘I like or dislike bananas’).
Because the metaphor is quite simple, it can be also extended to explain many facets of the human experience and how they relate to this probabilistic nature of the Bayesian brain:
1) Humans have intrinsic fears of certain animals, such as snakes and spiders, developed throughout the mammalian evolutionary history.11 For the belief “I like or I do not like spiders”, we could visualize that the scale is naturally tipped to the dislike side — meaning that, even without any experiential evidence, humans have an intrinsic fear of arachnids.
2) Humans have a salience bias, meaning that emotionally evocative information is more persuasive than neutral ones. For instance, when deciding for the belief ‘Planes are safe or unsafe’, one may conclude the latter based on shocking imagery they once saw on TV of a plane crash — even if statistics show that planes are objectively one of the safest modes of transportation.12 In the context of the metaphor, one may visualize that the evidence pebbles have an emotional component that gives them extra weight.
3) Humans tend to make judgments based on more recent information – which is a phenomenon known as the ‘availability heuristic’.13 For example, when deciding ‘Elevators are safe or unsafe’, one’s judgment might be severely skewed to the ‘unsafe’ side if they saw a coworker get stuck in an elevator two days before. In the scale metaphor, one could imagine that the pebbles of evidence wear out and become lighter over time, exerting less influence over the belief scale.
As far as disadvantages, the metaphor is fairly abstract in content, which means that it can be used to explain essentially anything – as exemplified in this article, in which I use it to explain food preferences, visual perception, and cognitive biases. This could be considered a strong weakness because it is often unclear how the individual elements of the metaphor relate to reality. The lack of clarity may induce misunderstandings among laypeople: do humans have millions of scales inside their heads, each one making one individual decision?
The metaphor also attempts to reduce all complexities of learning and decision-making into single inanimate objects. This not only can be viewed as an oversimplification of what occurs in the brain, but it implicates a hard deterministic interpretation of free will14: by stating that beliefs are explained by previous experiences and biological predispositions under the gaze of a purely physical process (placing weights on top of scales), the imagery strongly hints to a fully deterministic universe which does not leave space for the sense of one’s agency in modifying their beliefs.
Another strong weakness of the metaphor is that it does not account for the fact that beliefs are interdependent15: ‘I like or dislike bananas’ is not only dependent on one’s past experience, but also dependents on their current beliefs and feelings, such as ‘I like or dislike fruits in general’, ‘I am really hungry right now’ or ‘I am really tired and I can’t even think about food’. Maybe the metaphor could be extended to include that each ‘belief scale’ is converted into a pebble of evidence into another belief scale, or perhaps you place scales on top of scales to represent this interdependency – but it then becomes convoluted and unclear as an explanatory device.
It is worth noting that the metaphor can be vague in meaning because the hypothesis itself is also fairly abstract: the Bayesian Brain hypothesis has yielded many criticisms, including one of being unscientific16. The statement “The brain is performing probabilistic decisions like a computer” does not provide a mechanism by which the computations are performed and it is therefore unfalsifiable. Under a strict Popperian view of science, this would mean that the hypothesis is unscientific and therefore not worthy of any merit.
Science still does not have a definitive answer about fundamental processes underlying our decision making, and the Bayesian brain is just one hypothesis that tries to explain the disconnect between ‘why we cannot perceive reality’ and ‘how we operate in the environment’. The Bayesian brain has been put in vogue in the last decade, especially by computer scientists who use machine learning techniques that rely heavily on principles of Bayesian statistics17. However, just because computers use probabilistic statistics to make decisions, it does not follow that humans necessarily use the same mechanism – this would be an inductive fallacy of faulty generalization. The proponents of the Bayesian Brain hypothesis still need to demonstrate the existence of this underlying mechanism in humans.
Science communicators must be cautious in the way they explain science in popular media to properly separate what is established science from speculative hypotheses and personal opinions, especially in the field of Neuroscience, in which a lot of mysticism persists in the zeitgeist18. However, it also means that some of the most burning questions one could ask about the world are not scientific: no experiment could ever be devised to experimentally test what the true nature of reality is19. This begs the question: should we only focus on science to understand reality and forget about unfalsifiable hypotheses or should we be open to other forms of explaining how the world works?
To conclude, the use of metaphors is a commonly used device for explanations, because our brains have higher developed visual and language cortices, it is often easier to explain concepts in terms of vivid imagery and stories20,21; however, we need to be careful about the precision of our metaphors and possible misunderstandings they might generate. The scale metaphor provides an easy and generalizable way to understand the process of decision-making in the human brain, but it fails to account for the interdependence of beliefs and it diminishes one’s agency by appealing to purely physical processes.
References
1. Dale Purves, George Augustine, David Fitzpatrick, William Hall, Anthony-Samuel Lamantia, L. W. Neuroscience. 5th Edition. (Sinauer Associates, 2012).
2. Purves, D., & Lotto, R. B. Why we see what we do redux: a wholly empirical theory of vision. Choice Reviews Online 48, (Sinauer Associates., 2011).
3. Lübbig, H. & Helmholtz, H. von. The inverse problem : symposium ad memoriam Hermann von Helmholtz. Research, measurement, approval, xii, 225 p. (1995).
4. Darimont, C. T., Fox, C. H., Bryan, H. M. & Reimchen, T. E. The unique ecology of human predators. Science (80-. ). 349, 858–860 (2015).
5. Yuille, A. & Kersten, D. Vision as Bayesian inference: analysis by synthesis? Trends Cogn. Sci. 10, 301–308 (2006).
6. Wolpert, D. M. Probabilistic models in human sensorimotor control. Hum. Mov. Sci. 26, 511–524 (2007).
7. Pantelis, P. C. et al. Inferring the intentional states of autonomous virtual agents. Cognition 130, 360–379 (2014).
8. Houlsby, N. M. T. et al. Cognitive tomography reveals complex, task-independent mental representations. Curr. Biol. 23, 2169–2175 (2013).
9. Girshick, A. R., Landy, M. S. & Simoncelli, E. P. Cardinal rules: Visual orientation perception reflects knowledge of environmental statistics. Nat. Neurosci. 14, 926–932 (2011).
10. Wernery, J. et al. Temporal processing in bistable perception of the Necker cube. Perception 44, 157–168 (2015).
11. Öhman, A. & Mineka, S. Fears, phobias, and preparedness: Toward an evolved module of fear and fear learning. Psychol. Rev. 108, 483–522 (2001).
12. Slovic, P. The perception of risk. Sci. Mak. a Differ. One Hundred Eminent Behav. Brain Sci. Talk about their Most Important Contrib. 236, 179–182 (2016).
13. Schwarz, N. et al. Ease of Retrieval as Information: Another Look at the Availability Heuristic. J. Pers. Soc. Psychol. 61, 195–202 (1991).
14. Scheer, R. K. Free Will. Philosophical Investigations 13, (Free Press, 1990).
15. Glore, G. L. & Gasper, K. Feeling is believing: Some affective influences on belief. Emot. Beliefs 10–44 (2010). doi:10.1017/cbo9780511659904.002
16. Horgan, J. Are Brains Bayesian? Scientific American (2017). Available at: https://blogs.scientificamerican.com/cross-check/are-brains-bayesian/. (Accessed: 27th January 2020)
17. Bobrov, P. D., Korshakov, A. V., Roshchin, V. I. & Frolov, A. A. [Bayesian classifier for brain-computer interface based on mental representation of movements]. Zh. Vyssh. Nerv. Deiat. Im. I P Pavlova 62, 89–99 (2012).
18. O’Connor, C., Rees, G. & Joffe, H. Neuroscience in the Public Sphere. Neuron 74, 220–226 (2012).
19. Jackendoff, R. The Problem of Reality. Noûs 25, 411 (1991).
20. Exhibit, S., Batsak, B. & Orlov, O. Visual metaphor comprehension : an fMRI study. (2018).
21. Zadbood, A., Chen, J., Leong, Y. C., Norman, K. A. & Hasson, U. How We Transmit Memories to Other Brains: Constructing Shared Neural Representations Via Communication. Cereb. Cortex 27, 4988–5000 (2017).