Top-down control and goal-directed behavior: on the role of the medial prefrontal cortex (mPFC) in positive and negative valence paradigms
1 ABSTRACT
Despite being a very common term in Neuroscience research, a lot of ambiguity persists in the literature regarding the precise definition of top-down control. In this review, we propose a more rigorous model of ‘top-down control’ as the integration of information contingent upon the maturation of neuronal ensembles. This model is explored in negative and positive valence studies that have investigated the medial prefrontal cortex (mPFC), an important heteromodal association cortex that is related to goal-directed behavior. In face of the new definition, we conclude that the maturation of neuronal ensemble in the mPFC is necessary for goal-directed behavior. We posit that a focus on the mechanisms of ensemble maturation could become a unifying facet of future research around the mPFC, allowing different lines of neuroscientific investigation to contribute to one another.
2 TOP-DOWN CONTROL IN NEUROSCIENCE RESEARCH
The definition of ‘top-down’ and ‘bottom-up’ models has been widely adopted by many scientific fields, with different and often contradictory meanings amongst them. In the field of Neuroscience and Psychology, the term ‘top-down’ is commonly used as jargon in scientific papers but rarely actually defined (Rauss & Pourtois, 2013), which likely stems from a lack of consensus on a rigorous definition for top-down processing. As a result, the term is often used in contradictory ways. For instance, ‘top-down control’ has been used as a defining characteristic of the visual processing in V1 in anesthetized ferrets (Roland et al., 2006), of the stress-regulating influence of the mPFC over the thalamus-BNST-amygdala pathway in rodents (de Kloet, de Kloet, de Kloet, & de Kloet, 2019) and for the role the parietal cortex in attention orienting in primates (Shomstein, 2012) – the same term used for different species, different states of consciousness, different brain regions entirely. It could be argued that such a definition would be rendered useless due to its broadness in scope.
To have a more precise definition of top-down control in the context of Neuroscience, it is necessary to think in terms of hierarchies in information encoding. One of the fundamental functions of the nervous system is to perform information processing, taking complex environmental and interoceptive inputs and allowing the organism to perform actions in accordance with its environment – a process coined as the ‘perception-action cycle’ (Fuster, 2001). As the information flows from the peripheral nervous system to the spine to sensory cortices to association cortices, information is encoded via mechanisms that are intra-neuronal (e.g. changes in gene expression, receptor expression, and spine morphology) and extra-neuronal (e.g. myelin plasticity) (Tozzi, 2015). Changes in neuronal activity promote changes in neuronal connectivity, resulting in the formation of ‘neuronal ensembles’ or ‘memory traces’– biological substrates that encode a particular memory (Thompson, 2005).
We propose a definition of top-down and bottom-up processing as follows: at lower levels of the hierarchy (e.g. peripheral nervous system or PNS) bottom-up processing occurs and the information is processed at a greater level of detail. On the other hand, at higher levels of the hierarchy (e.g. association cortices), top-down processing takes place, meaning that the incoming information is integrated (Figure 1). What differentiates hierarchical levels is their relative sparse connectivity: the information encoded by many ensembles in lower hierarchical levels is condensed into fewer ensembles in higher hierarchical levels. The condensation of information is the characteristic that allows information to be integrated from multiple inputs in higher hierarchical levels. Importantly, this model does not pose that only frontal cortices exert top-down control (e.g. definition adopted by White et al., 2018). For example, sensory cortices may exert top-down control over afferent spinal inputs. Moreover, the same brain region can have top-down and bottom-up processing occurring simultaneously: for instance, a sensory cortex can exert top-down control over the spinal inputs while providing efferent bottom-up signals to an association cortex.
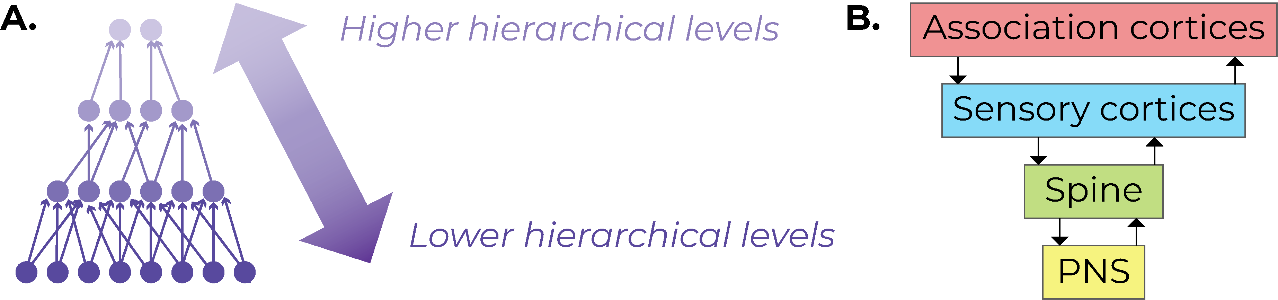
Critically, this definition differs from the general way it is used in literature: some authors have asserted that the activity of neocortical regions implies top-down control over subcortical structures (Chiesa, Serretti, & Jakobsen, 2012). Under our proposed definition, the mere simultaneous firing of a brain region at a higher hierarchical level with another region at a lower hierarchical level does not necessarily imply top-down control. Rather, top-down control occurs at an ensemble level, contingent upon changes in cellular activity and connectivity. Since brain regions at higher hierarchical levels (e.g. heteromodal association cortices) have sparse connections with many other brain regions, we propose that their capacity to integrate information does not occur immediately. Instead, the memory ensemble undergoes a process of maturation, in which the connections in higher hierarchical levels are gradually strengthened over a period of time (see Section 4.3).
3 GOAL-DIRECTED BEHAVIOR AND THE MPFC
As mammals evolved, their actions became more complex – i.e. based less on simple stimulus-response loops and contingent on prior experience (Carlén, 2017). The ability of an organism to appropriately modify its actions to optimize the possible outcomes in a given scenario has been coined as goal-directed behavior (Zwosta et al., 2015). Goal-directed behavior is uniquely different from innate reflexes or habitual actions because there is no predetermined set of actions which could be constructed ex-ante. Instead, the organism needs to promptly adapt its actions based on constantly changing environmental stimuli. (Verschure, Pennartz, & Pezzulo, 2014)
Goal-direction involves brain-wide networks and therefore no single brain region should be considered a ‘goal-direction center’ of the brain. However, the capacity to integrate multimodal forms of input is paramount for animals to behave sensibly to changes in their environment. Therefore, goal-direction is contingent upon the exertion of top-down control from associative cortices over sensory and limbic cortices (see Section 2). While other associative cortices, such as the parietal cortex (Cohen, 2009), have been related to goal-direction, this review will mainly focus on the medial prefrontal cortex (mPFC).
The mPFC can be subdivided into the dorsomedial prefrontal cortex (dmPFC), which constitutes the anterior cingulate (AC) and the most dorsal section of the prelimbic cortex; and the ventromedial prefrontal cortex (vmPFC), which constitutes the infralimbic cortex (IL) and the ventral-most section of the prelimbic cortex (Figure 2) (Uylings & Van Eden, 1991). The vmPFC receives more limbic projections and processes emotional and interoceptive information while the dmPFC has more connections with sensory and motor regions (Heidbreder & Groenewegen, 2003).
The mPFC integrates motor information, exogenous stimuli (incoming mainly from thalamus), and endogenous stimuli (incoming from connections with the limbic system, which includes the amygdala, hippocampus, and nucleus accumbens) (Kamigaki, 2019). Therefore, the mPFC is anatomically positioned to integrate multiple modes of information and to modulate behavior (Gazzaley & Nobre, 2012). This rich anatomical connectivity allows the mPFC to act as an important hub for goal-directed behaviors, allowing the association between certain actions to positive outcomes and others to negative outcomes, thereby increasing the organism’s adaptability over time. (Kamigaki, 2019)
In behavioral neuroscience, two broad types of paradigms can be used: Pavlovian or instrumental. In pavlovian conditioning, the animal learns an association between two stimuli (e.g. a sound and a food reward). In contrast, in instrumental conditioning, the animal associates a self-initiated behavior with a stimulus (e.g. a nose poke with a food reward). Furthermore, paradigms can also be defined by the valence of their stimuli: a stimulus can be appetitive if the outcome is a reward (e.g. a food reward) or aversive if the outcome is a punishment (e.g. a foot shock). The valence of the outcome is important because it primes the attention of the organism towards the context: it is often the case that a neutral outcome does not form a robust memory (Lonsdorf et al., 2017). Therefore, in both Pavlovian and instrumental setups, the valence of a stimulus (whether positive or negative) has an impact on the memory formed: in Pavlovian conditioning, the valence potentiates the association between both stimuli, while in instrumental conditioning, it modifies the likelihood of the animal performing the same behavior in the future.
The following sections are a discussion of two subtypes of study which have investigated the mPFC: Pavlovian-negative (focusing mainly on fear-conditioning paradigms) and Instrumental-positive (focusing on addiction studies and the delay-discounting task). It is important to note that they are extremes in terms of training complexity: fear conditioning might require only one session to establish a memory that will last for the entire lifetime of the animal (Gale et al., 2004), whereas training in the delay-discounting task might take several weeks (Robbins, 2007). Despite this striking difference, we will propose that ensemble maturation is a unifying characteristic of both types of study. We will describe the basic circuitry involved in positive and negative valence studies, followed by a delineation of the relationship between mPFC, top-down control, and goal-direction in each study type.
4 TOP-DOWN CONTROL IN NEGATIVE VALENCE STUDIES
4.1 INTRODUCTION TO FEAR CONDITIONING
The expression of fear offers evolutionary advantages for animals and can be construed as an aspect of goal-direction: the brain must associate environmental cues with negative valence stimuli, which allows the animal to adapt its behavior in a future encounter with the same environment in order to optimize possible outcomes. Moreover, this process of association has an element of uncertainty because no organism can encounter every possible environment. Instead, animals need to have a model of the world which is based on previous experiential evidence (Rusu & Pennartz, 2019). The organism not only learns which environments are safe or unsafe, but it also uses this information to inform the behavioral decisions upon encountering new and unknown environments. Furthermore, animals also need to be flexible and be able to extinct fear memories, because environments which were once threatening in the past may be safe in the future (Moscarello & Maren, 2018).
A paradigm devised to model this natural phenomenon and to explore the mechanisms of associative learning is fear conditioning. Fear conditioning involves the association of a neutral conditioned stimulus (CS) paired with an aversive unconditioned stimulus (US). The animal is placed in an operant box for the first time and it receives a foot shock a few minutes later – the pairing of CS and US is called fear acquisition. In auditory fear conditioning, the CS is a tone and in contextual fear conditioning, the CS is the contextual information. After fear acquisition, the animal is subsequently provided with the same CS, but this time it does not receive a foot shock – this process is named extinction training. Importantly, during extinction, the original fear memory is not erased, but rather a new competing memory is established (An et al., 2017), which explains why extinction training suppresses the fear memory only transiently (Bouton, 2004) and in a context-dependent manner (Bouton & Bolles, 1979). Posterior to extinction training, the animal is confronted with the original CS again to test if the fear memory is reinstated after the extinction phase – which is a process known as ‘renewal’. In all stages of fear conditioning, the animal’s freezing behavior is used as a proxy of the underlying fear memory.
The mPFC seems to be important in two moments of fear conditioning: renewal, which has been mostly associated with the PL, and extinction, which has mostly implicated the IL (Knapska & Maren, 2009; Stern, Gazarini, Vanvossen, Hames, & Bertoglio, 2014). For decades, with increasingly advancing methods, the goal of this field of research has been to unveil the underlying ‘fear circuitry’, which has been found to involve the interaction between the mPFC, amygdala, and hippocampus. (Maren, Phan, & Liberzon, 2013)
4.2 THE INTERPLAY BETWEEN AMYGDALA AND MPFC
The amygdala can be subdivided into two main nuclei: the basolateral amygdala (BLA), which constitutes the lateral, basal and basomedial nuclei; and the central nucleus (CeA), which constitutes lateral and medial subregions (Pitkänen, Savander, & LeDoux, 1997). The BLA receives sensory inputs via thalamus and it receives projections from neocortical structures, such as the hippocampus and the mPFC (Pitkänen, Pikkarainen, Nurminen, & Ylinen, 2006). The BLA has long been thought of as the site in which the pairing of CS and US would occur (Marek, Sun, & Sah, 2019), while the CeA has been considered the main output of conditioned fear responses. Connecting the BLA and CeA is a group of GABAergic neurons called intercalated cells (ITC) (Duvarci & Pare, 2014).
One of the first examples of the interaction between the mPFC and the amygdala in a fear conditioning paradigm was the observation that a lesion in the vmPFC induced impairments in extinction (Morgan, Romanski, & LeDoux, 1993). It has been later shown that in the course of extinction learning, there is a reduction in synaptic efficacy in glutamatergic neurons of the mPFC that project to principal neurons in the BLA; however, the synaptic efficacy of mPFC projections to ITCs remains unchanged, leading to enhanced inhibition of the central nucleus of the amygdala (Cho, Deisseroth, & Bolshakov, 2013). It has also been shown that extinction can be facilitated by the induction of synaptic depression of a monosynaptic projection between BLA and mPFC (Klavir, Prigge, Sarel, Paz, & Yizhar, 2017). Furthermore, optogenetically silencing projections from the IL to the BLA impairs extinction learning while optogenetically stimulation of this pathway enhances extinction learning. (Bukalo et al., 2015)
Although there is a bidirectional anatomical connection between mPFC and amygdala, this does not entail that the mPFC always exerts top-down control over the amygdala. For instance, the behavioral response of freezing itself, immediately after acquisition, is mainly driven by the amygdala and periaqueductal gray function (Herry & Johansen, 2014). The disruption of mPFC activity in the initial fear acquisition stage does not inhibit the freezing response (Gilmartin, Balderston, & Helmstetter, 2014; Heroux, Robinson-Drummer, Sanders, Rosen, & Stanton, 2017; Lee & Choi, 2012; Zelinski, Hong, Tyndall, Halsall, & McDonald, 2010), suggesting that the initial fear expression is not mediated by mPFC function.
However, the function of goal-direction of the mPFC in fear conditioning lays upon the fact that the expression of fear is not appropriate under all circumstances. Upon encountering a possibly threatening environment, the organism needs to recall the context in which it previously had negative experiences and then prime an adaptive response – i.e. to perceive the new context as high-threat and flee/freeze or to perceive it as low-threat and behave in a normal, exploratory way. The choice of behavior from a complex repertoire must be finely controlled and is not only based on current stimuli, but also on the possible imminent threats that could occur the next moment (Giustino & Maren, 2015). Therefore, the mPFC could be considered as a goal-direction hub in fear conditioning, not because of the expression of fear itself, but rather determining when to express fear as the most appropriate behavior. (Moscarello & Maren, 2018)
4.3 THE INTERPLAY BETWEEN HIPPOCAMPUS AND MPFC
The hippocampus is thought to provide contextual information, as evidenced by the fact that hippocampal lesions lead to impaired fear expression when a foot shock is paired with a context, but not when it is paired to an auditory tone (Phillips & LeDoux, 1992). More recent research has corroborated that idea, showing that hippocampal neurons are preferentially active during context presentation, independent of whether the animal is immediately shocked or previously fear conditioned. (Zelikowsky, Hersman, Chawla, Barnes, & Fanselow, 2014). Although the relationship between hippocampus and mPFC has long been thought as one of excitatory feed-forward excitation (Padilla-Coreano et al., 2016), recent evidence has suggested that more important for fear extinction is the feedforward inhibition of the hippocampus to PV-positive interneurons in the IL, but not somatostatin-positive interneurons or principal glutamatergic neurons in the IL (Marek et al., 2018).
Outside the field of fear conditioning studies, the interplay between hippocampus and mPFC has been traditionally been thought as one of the supporters of memory consolidation (Alvarez & Squire, 1994): the hippocampus and its surrounding entorhinal cortex would function as the neural substrates for recent memories while the mPFC and other neocortical regions would serve as substrates for remote memories (McClelland, 2013), although the exact mechanisms of systems consolidation remained elusive for a long time.
In a recent seminal paper, Kitamura et al. used ensemble-specific techniques to demonstrate a possible mechanism to the consolidation of fear memory in the mPFC over time. Rather than being initially encoded in the hippocampus and later transitioning to mPFC, they found that there were mPFC ensembles formed during the initial stage of fear acquisition (Kitamura et al., 2017). These mPFC ensembles were found to receive projections from both the hippocampus and the BLA, indicating that they could be sites of top-down control over the incoming bottom-up signals. These mPFC ensembles, however, were initially immature and were not naturally activated by environmental cues, but they could be artificially activated with optogenetics to induce freezing (Kitamura et al., 2017).
The authors also found an opposing effect of maturation between mPFC and hippocampus: they found that the initial immature mPFC ensemble became mature within 14 days, and the initially active hippocampal ensemble was no longer involved after 14 days. Meanwhile, BLA ensembles were persistent throughout the entire period, which suggests its role in encoding the valence of a fear memory (Kitamura et al., 2017). An interesting hypothesis to explain the transition between a hippocampal-driven memory and a neocortical-driven memory is that the hippocampus is constantly creating new neurons, which could integrate into the hippocampal network and disrupt established memory ensembles. (Kitamura & Inokuchi, 2014)
This study helps to answer an important question discussed previously (see Section 4.2): why is mPFC activity not important for the initial expression of fear, but rather the initial fear expression seems to be driven mainly by subcortical structures? One possible answer that the association of inputs from lower hierarchical levels (hippocampus and amygdala) is not initially strong enough to allow mPFC ensembles to exert top-down control over the primed bottom-up signals. These mPFC ensembles need time to become mature (Figure 3) and in the context of fear conditioning, this maturation takes around 14 days and results on increased complexity in dendritic morphology in these ensemble cells (Kitamura et al., 2017).
4.4 LIMITATIONS OF FEAR CONDITIONING
An important limitation of the fear conditioning paradigm is its dependence on fear and pain circuits (Herry & Johansen, 2014), which results in memory effects that are not generalizable to other forms of learning. This can be exemplified with the phenomenon of incubation, in which the fear response can be potentiated over time without extra training, which is a phenomenon known as incubation (Eysenck, 1968).
Furthermore, stress itself has been shown as a confounding factor in fear conditioning: exposure to chronic stress enhances fear expression (Maroun et al., 2013) and it is correlated with a decrease in dendritic morphological complexity in the mPFC. (Izquierdo, Wellman, & Holmes, 2006). A recent study has demonstrated that mild-fear conditioning promotes the formation of an ensemble in the mPFC, whose activation is sufficient to induce fear expression one month later. However, a strong fear conditioning (using three foot shocks, instead of one) does not induce the formation of mPFC ensembles (Matos et al., 2019).
5 TOP-DOWN CONTROL IN POSITIVE VALENCE STUDIES
In the field of positive valence paradigms, it has been consistently found that mPFC impairments do not necessarily decrease the performance of the animal in the tasks. However, mPFC impairments usually have a negative impact on aspects related to task switching and cognitive flexibility (Floresco, Block, & Tse, 2008). This general phenomenon relates to the importance of the mPFC in the integration of heteromodal information to allow goal-directed behavior.
Similar to negative valence studies, the term top-down control is often used in positive valence studies to describe the role of the mPFC in attention and impulsivity (Miller & D’Esposito, 2005). We posit that this general definition is not correct: instead, similarly to the presented evidence from aversive stimuli studies (see Section 4.3), top-down control should be mediated by mechanisms of ensemble maturation in the mPFC.
5.1 ADDICTION STUDIES
Addiction studies are an all-encompassing definition for paradigms that use addictive substances as positive valence stimuli. One example is the forced-abstinence paradigm, in which the animal goes through a period of training in which it associates an action (e.g. a lever press) with a drug reward. After this initial training period, the drug reward is paired with a negative stimulus (e.g. a foot shock). The goal of this type of study is to assess the control of impulsivity, observing if the animal is capable of refraining from a short-term reward (drug reward) because of its longer-term consequences (after a while, it will receive a foot shock as a punishment).
Using this type of paradigm, it has been shown that long-term cocaine self-administration reduces PL excitability, which can be rescued with optogenetic stimulation. (Chen et al., 2013) This suggests that hypoactivity of mPFC is related to a loss of top-down control and consequent compulsive drug seeking. Furthermore, a recent study has shown that when pairing a lever press to a punishment, there is a notable shift in synaptic plasticity in the vmPFC neurons which project to the shell of the nucleus accumbens (NAc) (Halladay et al., 2020).
While the amygdala has a very clear role in encoding ‘fear memories’ in the context of negative valence paradigms, it has also been implicated in the processing of positive valence stimuli. An increase in neuronal firing of the BLA is necessary for the formation of associative reward memories (Tye, Stuber, De Ridder, Bonci, & Janak, 2008) and BLA neurons respond to both reward and punishment in a pavlovian task with distinct underlying neuronal populations (Beyeler et al., 2016). Although no anatomical distinction seems to exist within the amygdala to separate ensembles that encode positive or negative valence stimuli, there are molecular markers for the encoding of valence. In particular, magnocellular Rspo2+ neurons are mainly activated by aversive stimuli while parvocellular Ppp1r1b+ neurons are mainly activated by appetitive stimuli (Kim, Pignatelli, Xu, Itohara, & Tonegawa, 2016), which suggests a molecular substrate for how the BLA could encode two antagonistic types of memory.
Classically, the vmPFC has been shown as a center for inhibitory control over drug-seeking (Moorman, James, McGlinchey, & Aston-Jones, 2015) and the dmPFC has been thought to drive drug-seeking behavior. This idea of dmPFC as a ‘go’ center and vmPFC as a ‘no-go’ center has an obvious parallel with fear conditioning studies, in which the dmPFC is related to fear expression and vmPFC is related to extinction learning (Giustino & Maren, 2015). However, a more nuanced view is necessary to understand mPFC function. For example, IL activation can either induce increase or decrease in drug-seeking (Koya et al., 2009; Peters, LaLumiere, & Kalivas, 2008), which could potentially be explained by a more complex time-dependent function of the vmPFC in the expression and extinction of cocaine-seeking (Van den Oever et al., 2013).
Cocaine self-administration results in enhancement of excitatory activity in the PL-to-NAc(core) pathway, while extinction results in an increase of excitation in the IL-to-NAc(shell) pathway. Both enhancements of excitation are mediated by synaptic maturation through the upregulation of AMPA receptors and optogenetic inhibition of the synaptic remodeling process results in a decrease of drug-seeking (Ma et al., 2014). While this study did not use ensemble-specific targeting, the evidence related to synaptic modeling with drug craving could fall in line with the proposed idea that the exertion of top-down processing of the mPFC depends on mature memory traces.
To direct assess ensemble function in addiction, a recent study has used the method of targeted recombination of active populations (TRAP) to specifically tag neurons that were naturally active during an alcohol self-administration task. The authors found that a small mPFC ensemble was necessary for cue-induced alcohol-seeking but not necessary for context-induced alcohol-seeking (i.e. when a salient cue was not present in the testing stage) (Visser et al., 2020). Furthermore, chemogenetic inactivation of the small alcohol-associated ensemble in the mPFC (6-7% of total neurons) led to a decrease in cue-induced alcohol-seeking, while inactivation of similarly sized sucrose-related ensemble did not lead to the same effect, which specifically demonstrates the effected of inactivation of the memory ensemble related to drug-seeking (Visser et al., 2020).
An important limitation of studies that use highly addictive drugs as rewards is the demonstration that cocaine-seeking becomes insensitive to devaluation after extensive training, which means that the behavior was not goal-directed, but rather habitual (Zapata, Minney, & Shippenberg, 2010). Therefore, in order to investigate goal-directed behavior, potentially a paradigm be used with two differences: (1) a less potent rewarding stimulus and (2) a task that has variance in the stimulus-response setups, such that an automatized pattern of behavior emerges from the mPFC.
5.2 DELAY-DISCOUNTING TASK (DDT)
Impulsivity can be defined as a premature action without foresight (Dalley, Everitt, & Robbins, 2011). The relationship between different brain regions and impulsivity has been studied for decades with paradigms such as the delay discounting task (DDT). In the DDT, the animal is trained to perform an action to receive a small reward (e.g. one food pellet) or wait for a few seconds and perform the same action to receive a large reward (e.g. five food pellets). One important methodological consideration is that the animal is unable to receive more rewards by performing a series of sequential short-term actions, therefore a time buffer needs to be put in place in between trials (Beckwith, 2017).
DDT is a powerful paradigm to assess a straightforward aspect of goal-direction. This paradigm has a reduction in confounding factors because both choices (short/small or long/large) have the same patterns of behavioral output, i.e. a waiting period followed by the same movement, which is different from the 5-choice serial-reaction time task, for example, where some responses require more movement than others. This is of extreme importance to study the mPFC because the dmPFC is also involved in motor planning (Euston & McNaughton, 2006). Therefore, in studying the waiting periods in the DDT, researchers can assess mPFC activity related to the choice of the animal, i.e. higher-order cognitive function without motor planning as a confounder.
In the DDT, pharmacological inactivation or neurotoxic lesions of the mPFC and disconnection between mPFC-BLA led to increased impulsivity (preference of short-term small reward over long-term big rewards) (Churchwell, Morris, Heurtelou, & Kesner, 2009; Gill, Castaneda, & Janak, 2010), This increase in impulsivity indicates a role in the mPFC of representing the outcome values of certain behavioral responses, which has later demonstrated with electrophysiological studies (Powell & Redish, 2016). In particular, dmPFC neurons are more active during the delay stage of the DDT and pharmacological inactivation of this brain region increases premature responses (Narayanan & Laubach, 2006).
Interestingly, electrophysiological recordings revealed an increase in PL activity during the delay period of a large reward, however, this increase was not observed when the animals were performing forced-choice trials (Sackett, Moschak, & Carelli, 2019). This parallels findings in habitual behavior research, where mPFC function is generally important for the learning stages of a new task, but once the contingencies of the task are learned, the mPFC becomes disengaged and behavior is driven mainly by subcortical structures, such as the dorsal striatum (Everitt & Robbins, 2016).
To date, no studies have investigated the mPFC using ensemble-specific targeting. A potential prolific field of research would investigate the development of ensemble maturation in the mPFC during the initial learning stages of an instrumental task up to when the actions of the animal become habitual, i.e. at which points of learning a complex task does the mPFC exert top-down control and at which points it does not.
6 CONCLUSIONS AND PERSPECTIVES FOR THE FIELD
In this review, we proposed a model of top-down and bottom-up as a hierarchy of sparse connectivity. This model, which places neuronal ensemble maturation as paramount for the function of top-down processing in the nervous system, could be a catalyzer for the integration of multiple lines of research, including negative and positive valence studies. In the mPFC, this entails that top-down control is necessary for goal-directed behavior, as evidenced from positive and negative valence paradigm studies, although the underlying process of maturation depends on the specific parameters of the task. Importantly, the presented evidence does not entail that the mPFC is the only region related to goal-directed behavior or that can exert top-down control. The role of the mPFC is an instantiation of the importance of association cortices in the integration of information from lower hierarchical structures. It is possible that ensemble maturation in other association cortices, such as the parietal cortex (Ivashkina, Gruzdeva, Roshchina, Toropova, & Anokhin, 2019), or even in a cortex-wide dispersed-ensemble fashion (Roy et al., 2019).
As for methodological considerations, the mPFC is a complex heteromodal associative cortex and its function not only depends on the task at hand, but it is also influenced by all previous experiential learnings of the animal throughout its life (Tse et al., 2007). Careful interpretation of results must be done to avoid problems such as the Euston-Cowen-McNaughton hassle, in which dmPFC activity encodes mainly motoric activity rather than cognitive function in a task where the animal is moving. Experimental design to study the mPFC would benefit from either: 1) using paradigms that have the same output response in both task contingencies (like the DDT) and; 2) use analysis of video recordings to try to unveil mPFC activity which is related to movement and mPFC activity related to the task (Mathis et al., 2018).
Furthermore, mPFC function is disrupted through stress, possibly mediated by the upregulation of dopaminergic and noradrenergic receptors, which would enhance calcium-cAMP pathways and ultimately would result in a reduction of mPFC activity and a subsequent reduction in connectivity of the ensembles (Datta & Arnsten, 2019). Therefore, standardization of protocols across labs and advances in technologies that minimize contact between researchers and animals to reduce stress, such as operant chambers connected to the home cage of the animal (Bruinsma et al., 2019), would be useful to reduce confounding effects and possibly contradictory findings in the field.
Another consideration is the fact that, under unchanging environmental conditions, learning becomes habitual, i.e. less dependent upon neocortical activity and more dependent on subcortical activity (Everitt & Robbins, 2016), indicating a loss of top-control of higher hierarchical structures. A useful experiment to elucidate the relationship between habits and ensemble development in the mPFC would be to use fos-Cre-GCaMP to visualize ensemble activity in the mPFC during the learning stages complex instrumental paradigm.
The main reason why ensemble-specific studies are powerful is their reduction of uncertainty in the interpretation of data. This is especially true for association cortices like the mPFC, which is integrated with motor, limbic and sensory cortices. In this brain region, the general manipulation of neurons via optogenetics or chemogenetics might induce downstream changes in neuronal activity which are unrelated to the task at hand. In specific targeting engrams, these confounding effects are reduced and the subsequent change in behavior can be interpreted more precisely.
Recent efforts have tried to unveil the intracellular and extracellular mechanisms of neuronal maturation. In terms of intracellular mechanisms, consolidation of fear memory requires BNDF upregulation in the PL, which leads to the increase in neuroligin 1 (NLGN1) and neuroligin 2 (NLGN2), important markers of synaptic maturation (Ye, Kapeller-Libermann, Travaglia, Inda, & Alberini, 2017). As for extracellular mechanisms, myelin plasticity seems to be important for fear learning: in transgenic animals that cannot produce myelin, there is a deficiency in remote fear memory recall. This phenotype can be partially rescued by the induction of myelin expression in the brain (Pan, Mayoral, Choi, Chan, & Kheirbek, 2020).
To conclude, a focus of future research on unveiling the specifics of ensemble maturation may yield fruitful results and allow the cross-communication between various lines of neuroscience research. Further research is still needed to assess: 1) how the valence and strength of a stimulus are related to ensemble maturation in the learning of different paradigms 2) what is the interplay between changes in vasculature, myelination, synaptic morphology and protein expression in the process of ensemble maturation.
7 REFERENCES
Alvarez, P., & Squire, L. R. (1994). Memory consolidation and the medial temporal lobe: A simple network model. Proceedings of the National Academy of Sciences of the United States of America, 91(15), 7041–7045. https://doi.org/10.1073/pnas.91.15.7041
An, B., Kim, J., Park, K., Lee, S., Song, S., & Choi, S. (2017). Amount of fear extinction changes its underlying mechanisms. ELife, 6. https://doi.org/10.7554/eLife.25224
Beckwith, S. W. (2017). The Role of the Medial Prefrontal Cortex in Delay Discounting.
Beyeler, A., Namburi, P., Glober, G. F., Simonnet, C., Calhoon, G. G., Conyers, G. F., … Tye, K. M. (2016). Divergent Routing of Positive and Negative Information from the Amygdala during Memory Retrieval. Neuron, 90(2), 348–361. https://doi.org/10.1016/j.neuron.2016.03.004
Bouton, M. E. (2004, September). Context and behavioral processes in extinction. Learning and Memory. Learn Mem. https://doi.org/10.1101/lm.78804
Bouton, M. E., & Bolles, R. C. (1979). Contextual control of the extinction of conditioned fear. Learning and Motivation, 10(4), 445–466. https://doi.org/10.1016/0023-9690(79)90057-2
Bruinsma, B., Terra, H., de Kloet, S. F., Luchicchi, A., Timmerman, A. J., Remmelink, E., … Mansvelder, H. D. (2019). An automated home-cage-based 5-choice serial reaction time task for rapid assessment of attention and impulsivity in rats. Psychopharmacology, 1–12. https://doi.org/10.1007/s00213-019-05189-0
Bukalo, O., Pinard, C. R., Silverstein, S., Brehm, C., Hartley, N. D., Whittle, N., … Holmes, A. (2015). Prefrontal inputs to the amygdala instruct fear extinction memory formation. Science Advances, 1(6), e1500251. https://doi.org/10.1126/sciadv.1500251
Carlén, M. (2017, October 27). What constitutes the prefrontal cortex? Science. American Association for the Advancement of Science. https://doi.org/10.1126/science.aan8868
Chen, B. T., Yau, H. J., Hatch, C., Kusumoto-Yoshida, I., Cho, S. L., Hopf, F. W., & Bonci, A. (2013). Rescuing cocaine-induced prefrontal cortex hypoactivity prevents compulsive cocaine seeking. Nature, 496(7445), 359–362. https://doi.org/10.1038/nature12024
Chiesa, A., Serretti, A., & Jakobsen, J. C. (2012). Mindfulness: Top-down or bottom-up emotion regulation strategy? https://doi.org/10.1016/j.cpr.2012.10.006
Cho, J. H., Deisseroth, K., & Bolshakov, V. Y. (2013). Synaptic encoding of fear extinction in mPFC-amygdala circuits. Neuron, 80(6), 1491–1507. https://doi.org/10.1016/j.neuron.2013.09.025
Churchwell, J. C., Morris, A. M., Heurtelou, N. M., & Kesner, R. P. (2009). Interactions Between the Prefrontal Cortex and Amygdala During Delay Discounting and Reversal. Behavioral Neuroscience, 123(6), 1185–1196. https://doi.org/10.1037/a0017734
Cohen, Y. E. (2009). Multimodal activity in the parietal cortex. Hear Res, 258(2), 100–105. https://doi.org/10.1016/j.heares.2009.01.011
Dalley, J. W., Everitt, B. J., & Robbins, T. W. (2011, February 24). Impulsivity, Compulsivity, and Top-Down Cognitive Control. Neuron. Cell Press. https://doi.org/10.1016/j.neuron.2011.01.020
Datta, D., & Arnsten, A. F. T. (2019). Loss of prefrontal cortical higher cognition with uncontrollable stress: Molecular mechanisms, changes with age, and relevance to treatment. Brain Sciences, 9(5), 1–16. https://doi.org/10.3390/brainsci9050113
de Kloet, E. R., de Kloet, S. F., de Kloet, C. S., & de Kloet, A. D. (2019). Top-down and bottom-up control of stress-coping. Journal of Neuroendocrinology, 31(3), 1–16. https://doi.org/10.1111/jne.12675
Duvarci, S., & Pare, D. (2014, June 4). Amygdala microcircuits controlling learned fear. Neuron. Cell Press. https://doi.org/10.1016/j.neuron.2014.04.042
Euston, D. R., & McNaughton, B. L. (2006). Apparent encoding of sequential context in rat medial prefrontal cortex is accounted for by behavioral variability. Journal of Neuroscience, 26(51), 13143–13155. https://doi.org/10.1523/JNEUROSCI.3803-06.2006
Everitt, B. J., & Robbins, T. W. (2016). Drug Addiction: Updating Actions to Habits to Compulsions Ten Years On. Annual Review of Psychology, 67(1), 23–50. https://doi.org/10.1146/annurev-psych-122414-033457
Eysenck, H. J. (1968). A theory of the incubation of anxiety/fear responses. Behaviour Research and Therapy, 6(3), 309–321. https://doi.org/10.1016/0005-7967(68)90064-8
Floresco, S. B., Block, A. E., & Tse, M. T. L. (2008). Inactivation of the medial prefrontal cortex of the rat impairs strategy set-shifting, but not reversal learning, using a novel, automated procedure. Behavioural Brain Research, 190(1), 85–96. https://doi.org/10.1016/j.bbr.2008.02.008
Fuster, J. M. (2001). Prefrontal Cortex. In International Encyclopedia of the Social & Behavioral Sciences (pp. 11969–11976). https://doi.org/10.1016/B0-08-043076-7/03465-3
Gale, G. D., Anagnostaras, S. G., Godsil, B. P., Mitchell, S., Nozawa, T., Sage, J. R., … Fanselow, M. S. (2004). Role of the Basolateral Amygdala in the Storage of Fear Memories across the Adult Lifetime of Rats. Journal of Neuroscience, 24(15), 3810–3815. https://doi.org/10.1523/JNEUROSCI.4100-03.2004
Gazzaley, A., & Nobre, A. C. (2012, February). Top-down modulation: Bridging selective attention and working memory. Trends in Cognitive Sciences. NIH Public Access. https://doi.org/10.1016/j.tics.2011.11.014
Gill, T. M., Castaneda, P. J., & Janak, P. H. (2010). Dissociable roles of the medial prefrontal cortex and nucleus accumbens core in goal-directed actions for differential reward magnitude. Cerebral Cortex, 20(12), 2884–2899. https://doi.org/10.1093/cercor/bhq036
Gilmartin, M. R., Balderston, N. L., & Helmstetter, F. J. (2014, August). Prefrontal cortical regulation of fear learning. Trends in Neurosciences. Elsevier Ltd. https://doi.org/10.1016/j.tins.2014.05.004
Giustino, T. F., & Maren, S. (2015). The role of the medial prefrontal cortex in the conditioning and extinction of fear. Frontiers in Behavioral Neuroscience, 9(NOVEMBER). https://doi.org/10.3389/fnbeh.2015.00298
Halladay, L. R., Kocharian, A., Piantadosi, P. T., Authement, M. E., Lieberman, A. G., Spitz, N. A., … Holmes, A. (2020). Prefrontal Regulation of Punished Ethanol Self-administration. Biological Psychiatry, (28), 1–12. https://doi.org/10.1016/j.biopsych.2019.10.030
Heidbreder, C. A., & Groenewegen, H. J. (2003). The medial prefrontal cortex in the rat: evidence for a dorso-ventral distinction based upon functional and anatomical characteristics. https://doi.org/10.1016/j.neubiorev.2003.09.003
Heroux, N. A., Robinson-Drummer, P. A., Sanders, H. R., Rosen, J. B., & Stanton, M. E. (2017). Differential involvement of the medial prefrontal cortex across variants of contextual fear conditioning. Learning and Memory, 24(8), 322–330. https://doi.org/10.1101/lm.045286.117
Herry, C., & Johansen, J. P. (2014, January 1). Encoding of fear learning and memory in distributed neuronal circuits. Nature Neuroscience. Nature Publishing Group. https://doi.org/10.1038/nn.3869
Ivashkina, O., Gruzdeva, A., Roshchina, M., Toropova, K., & Anokhin, K. (2019). Ex vivo and in vivo imaging of mouse parietal association cortex activity in episodes of cued fear memory formation and retrieval. BioRxiv. https://doi.org/10.1101/863589
Izquierdo, A., Wellman, C. L., & Holmes, A. (2006). Brief uncontrollable stress causes dendritic retraction in infralimbic cortex and resistance to fear extinction in mice. Journal of Neuroscience, 26(21), 5733–5738. https://doi.org/10.1523/JNEUROSCI.0474-06.2006
Kamigaki, T. (2019, March 1). Prefrontal circuit organization for executive control. Neuroscience Research. Elsevier Ireland Ltd. https://doi.org/10.1016/j.neures.2018.08.017
Kim, J., Pignatelli, M., Xu, S., Itohara, S., & Tonegawa, S. (2016). Antagonistic negative and positive neurons of the basolateral amygdala. Nature Neuroscience, 19(12), 1636–1646. https://doi.org/10.1038/nn.4414
Kitamura, T., & Inokuchi, K. (2014, February 19). Role of adult neurogenesis in hippocampal-cortical memory consolidation. Molecular Brain. BioMed Central Ltd. https://doi.org/10.1186/1756-6606-7-13
Kitamura, T., Ogawa, S. K., Roy, D. S., Okuyama, T., Morrissey, M. D., Smith, L. M., … Tonegawa, S. (2017). Engrams and circuits crucial for systems consolidation of a memory. Science, 356(6333), 73–78. https://doi.org/10.1126/science.aam6808
Klavir, O., Prigge, M., Sarel, A., Paz, R., & Yizhar, O. (2017). Manipulating fear associations via optogenetic modulation of amygdala inputs to prefrontal cortex. Nature Neuroscience, 20(6), 836–844. https://doi.org/10.1038/nn.4523
Knapska, E., & Maren, S. (2009). Reciprocal patterns of c-Fos expression in the medial prefrontal cortex and amygdala after extinction and renewal of conditioned fear. Learning and Memory, 16(8), 486–493. https://doi.org/10.1101/lm.1463909
Koya, E., Golden, S. A., Harvey, B. K., Guez-Barber, D. H., Berkow, A., Simmons, D. E., … Hope, B. T. (2009). Targeted disruption of cocaine-activated nucleus accumbens neurons prevents context-specific sensitization. Nature Neuroscience, 12(8), 1069–1073. https://doi.org/10.1038/nn.2364
Lee, Y. K., & Choi, J.-S. (2012). Inactivation of the Medial Prefrontal Cortex Interferes with the Expression But Not the Acquisition of Diff erential Fear Conditioning in Rats. Experimental Neurobiology, 21(1), 23–29. https://doi.org/10.5607/en.2012.21.1.23
Lonsdorf, T. B., Menz, M. M., Andreatta, M., Fullana, M. A., Golkar, A., Haaker, J., … Merz, C. J. (2017). Don’t fear ‘fear conditioning’: Methodological considerations for the design and analysis of studies on human fear acquisition, extinction, and return of fear. Neuroscience and Biobehavioral Reviews, 77, 247–285. https://doi.org/10.1016/j.neubiorev.2017.02.026
Ma, Y. Y., Lee, B. R., Wang, X., Guo, C., Liu, L., Cui, R., … Dong, Y. (2014). Bidirectional modulation of incubation of cocaine craving by silent synapse-based remodeling of prefrontal cortex to accumbens projections. Neuron, 83(6), 1453–1467. https://doi.org/10.1016/j.neuron.2014.08.023
Marek, R., Jin, J., Goode, T. D., Giustino, T. F., Wang, Q., Acca, G. M., … Sah, P. (2018). Hippocampus-driven feed-forward inhibition of the prefrontal cortex mediates relapse of extinguished fear. Nature Neuroscience, 21(3), 384–392. https://doi.org/10.1038/s41593-018-0073-9
Marek, R., Sun, Y., & Sah, P. (2019, January 1). Neural circuits for a top-down control of fear and extinction. Psychopharmacology. Springer Verlag. https://doi.org/10.1007/s00213-018-5033-2
Maren, S., Phan, K. L., & Liberzon, I. (2013, June). The contextual brain: Implications for fear conditioning, extinction and psychopathology. Nature Reviews Neuroscience. https://doi.org/10.1038/nrn3492
Maroun, M., Ioannides, P. J., Bergman, K. L., Kavushansky, A., Holmes, A., & Wellman, C. L. (2013). Fear extinction deficits following acute stress associate with increased spine density and dendritic retraction in basolateral amygdala neurons. European Journal of Neuroscience, 38(4), 2611–2620. https://doi.org/10.1111/ejn.12259
Mathis, A., Mamidanna, P., Cury, K. M., Abe, T., Murthy, V. N., Mathis, M. W., & Bethge, M. (2018). DeepLabCut: markerless pose estimation of user-defined body parts with deep learning. Nature Neuroscience, 21(9), 1281–1289. https://doi.org/10.1038/s41593-018-0209-y
Matos, M. R., Visser, E., Kramvis, I., van der Loo, R. J., Gebuis, T., Zalm, R., … van den Oever, M. C. (2019). Memory strength gates the involvement of a CREB-dependent cortical fear engram in remote memory. Nature Communications, 10(1), 1–11. https://doi.org/10.1038/s41467-019-10266-1
McClelland, J. L. (2013). Incorporating rapid neocortical learning of new schema-consistent information into complementary learning systems theory. Journal of Experimental Psychology: General, 142(4), 1190–1210. https://doi.org/10.1037/a0033812
Mcklveen, J. M., Myers, B., Herman, J. P., & Herman, J. P. (2015). The Medial Prefrontal Cortex: Coordinator of Autonomic, Neuroendocrine and Behavioural Responses to Stress. Journal of Neuroendocrinology, 27, 446–456. https://doi.org/10.1111/jne.12272
Miller, B. T., & D’Esposito, M. (2005). Searching for “the top” in top-down control. Neuron, 48(4), 535–538. https://doi.org/10.1016/j.neuron.2005.11.002
Moorman, D. E., James, M. H., McGlinchey, E. M., & Aston-Jones, G. (2015). Differential roles of medial prefrontal subregions in the regulation of drug seeking. Brain Research, 1628(Pt A), 130–146. https://doi.org/10.1016/j.brainres.2014.12.024
Morgan, M. A., Romanski, L. M., & LeDoux, J. E. (1993). Extinction of emotional learning: Contribution of medial prefrontal cortex. Neuroscience Letters, 163(1), 109–113. https://doi.org/10.1016/0304-3940(93)90241-C
Moscarello, J. M., & Maren, S. (2018). Flexibility in the face of fear: hippocampal–prefrontal regulation of fear and avoidance. Current Opinion in Behavioral Sciences, 19, 44–49. https://doi.org/10.1016/j.cobeha.2017.09.010
Narayanan, N. S., & Laubach, M. (2006). Top-Down Control of Motor Cortex Ensembles by Dorsomedial Prefrontal Cortex. Neuron, 52(5), 921–931. https://doi.org/10.1016/j.neuron.2006.10.021
Padilla-Coreano, N., Bolkan, S. S., Pierce, G. M., Blackman, D. R., Hardin, W. D., Garcia-Garcia, A. L., … Gordon, J. A. (2016). Direct Ventral Hippocampal-Prefrontal Input Is Required for Anxiety-Related Neural Activity and Behavior. Neuron, 89(4), 857–866. https://doi.org/10.1016/j.neuron.2016.01.011
Pan, S., Mayoral, S. R., Choi, H. S., Chan, J. R., & Kheirbek, M. A. (2020). Preservation of a remote fear memory requires new myelin formation. Nature Neuroscience, 23(4), 487–499. https://doi.org/10.1038/s41593-019-0582-1
Peters, J., LaLumiere, R. T., & Kalivas, P. W. (2008). Infralimbic prefrontal cortex is responsible for inhibiting cocaine seeking in extinguished rats. Journal of Neuroscience, 28(23), 6046–6053. https://doi.org/10.1523/JNEUROSCI.1045-08.2008
Phillips, R. G., & LeDoux, J. E. (1992). Differential Contribution of Amygdala and Hippocampus to Cued and Contextual Fear Conditioning. Behavioral Neuroscience, 106(2), 274–285. https://doi.org/10.1037/0735-7044.106.2.274
Pitkänen, A., Pikkarainen, M., Nurminen, N., & Ylinen, A. (2006). Reciprocal Connections between the Amygdala and the Hippocampal Formation, Perirhinal Cortex, and Postrhinal Cortex in Rat: A Review. Annals of the New York Academy of Sciences, 911(1), 369–391. https://doi.org/10.1111/j.1749-6632.2000.tb06738.x
Pitkänen, A., Savander, V., & LeDoux, J. E. (1997). Organization of intra-amygdaloid circuitries in the rat: An emerging framework for understanding functions of the amygdala. Trends in Neurosciences, 20(11), 517–523. https://doi.org/10.1016/S0166-2236(97)01125-9
Powell, N. J., & Redish, A. D. (2016). Representational changes of latent strategies in rat medial prefrontal cortex precede changes in behaviour. Nature Communications, 7. https://doi.org/10.1038/ncomms12830
Rauss, K., & Pourtois, G. (2013). What is bottom-up and what is top-down in predictive coding. Frontiers in Psychology, 4(MAY), 276. https://doi.org/10.3389/fpsyg.2013.00276
Robbins, T. W. (2007). Delay Discounting and Impulsive Choice. Neuroscience, 1–18.
Roland, P. E., Hanazawa, A., Undeman, C., Eriksson, D., Tompa, T., Nakamura, H., … Ahmed, B. (2006). Cortical feedback depolarization waves: A mechanism of top-down influence on early visual areas. Proceedings of the National Academy of Sciences of the United States of America, 103(33), 12586–12591. https://doi.org/10.1073/pnas.0604925103
Roy, D. S., Park, Y.-G., Ogawa, S. K., Cho, J. H., Choi, H., Kamensky, L., … Tonegawa, S. (2019). Brain-wide mapping of contextual fear memory engram ensembles supports the dispersed engram complex hypothesis. BioRxiv. https://doi.org/10.1101/668483
Rusu, S. I., & Pennartz, C. M. A. (2019, January 1). Learning, memory and consolidation mechanisms for behavioral control in hierarchically organized cortico-basal ganglia systems. Hippocampus. John Wiley and Sons Inc. https://doi.org/10.1002/hipo.23167
Sackett, D. A., Moschak, T. M., & Carelli, R. M. (2019). Prelimbic cortical neurons track preferred reward value and reflect impulsive choice during delay discounting behavior. Journal of Neuroscience, 39(16), 3108–3118. https://doi.org/10.1523/JNEUROSCI.2532-18.2019
Shomstein, S. (2012). Cognitive functions of the posterior parietal cortex: Top-down and bottom-up attentional control. Frontiers in Integrative Neuroscience, (JULY 2012). https://doi.org/10.3389/fnint.2012.00038
Stern, C. A. J., Gazarini, L., Vanvossen, A. C., Hames, M. S., & Bertoglio, L. J. (2014). Activity in prelimbic cortex subserves fear memory reconsolidation over time. Learning and Memory, 21(1), 14–20. https://doi.org/10.1101/lm.032631.113
Thompson, R. F. (2005). In Search of Memory Traces. Annual Review of Psychology, 56(1), 1–23. https://doi.org/10.1146/annurev.psych.56.091103.070239
Tozzi, A. (2015, October 22). Information processing in the CNS: a supramolecular chemistry? Cognitive Neurodynamics. Kluwer Academic Publishers. https://doi.org/10.1007/s11571-015-9337-1
Tse, D., Langston, R. F., Kakeyama, M., Bethus, I., Spooner, P. A., Wood, E. R., … Morris, R. G. M. (2007). Schemas and memory consolidation. Science, 316(5821), 76–82. https://doi.org/10.1126/science.1135935
Tye, K. M., Stuber, G. D., De Ridder, B., Bonci, A., & Janak, P. H. (2008). Rapid strengthening of thalamo-amygdala synapses mediates cue-reward learning. Nature, 453(7199), 1253–1257. https://doi.org/10.1038/nature06963
Uylings, H. B. M., & Van Eden, C. G. (1991). Chapter 3 Qualitative and quantitative comparison of the prefrontal cortex in rat and in primates, including humans. Progress in Brain Research, 85(C), 31–62. https://doi.org/10.1016/S0079-6123(08)62675-8
Van den Oever, M. C., Rotaru, D. C., Heinsbroek, J. A., Gouwenberg, Y., Deisseroth, K., Stuber, G. D., … Smit, A. B. (2013). Ventromedial prefrontal cortex pyramidal cells have a temporal dynamic role in recall and extinction of cocaine-associated memory. Journal of Neuroscience, 33(46), 18225–18233. https://doi.org/10.1523/JNEUROSCI.2412-13.2013
Verschure, P. F. M. J., Pennartz, C. M. A., & Pezzulo, G. (2014). The why, what, where, when and how of goal-directed choice: Neuronal and computational principles. Philosophical Transactions of the Royal Society B: Biological Sciences, 369(1655). https://doi.org/10.1098/rstb.2013.0483
Visser, E., Matos, M. R., Van Der Loo, R. J., Marchant, N. J., De Vries, T. J., Smit, A. B., & Van Den Oever, M. C. (2020). A persistent alcohol cue memory trace drives relapse to alcohol seeking after prolonged abstinence. Retrieved from http://advances.sciencemag.org/
White, M. G., Panicker, M., Mu, C., Carter, A. M., Roberts, B. M., Dharmasri, P. A., & Mathur, B. N. (2018). Anterior Cingulate Cortex Input to the Claustrum Is Required for Top-Down Action Control. Cell Reports, 22(1), 84–95. https://doi.org/10.1016/j.celrep.2017.12.023
Ye, X., Kapeller-Libermann, D., Travaglia, A., Inda, M. C., & Alberini, C. M. (2017). Direct dorsal hippocampal-prelimbic cortex connections strengthen fear memories. Nature Neuroscience, 20(1), 52–61. https://doi.org/10.1038/nn.4443
Zapata, A., Minney, V. L., & Shippenberg, T. S. (2010). Shift from goal-directed to habitual cocaine seeking after prolonged experience in rats. Journal of Neuroscience, 30(46), 15457–15463. https://doi.org/10.1523/JNEUROSCI.4072-10.2010
Zelikowsky, M., Hersman, S., Chawla, M. K., Barnes, C. A., & Fanselow, M. S. (2014). Neuronal ensembles in amygdala, hippocampus, and prefrontal cortex track differential components of contextual fear. Journal of Neuroscience, 34(25), 8462–8466. https://doi.org/10.1523/JNEUROSCI.3624-13.2014
Zelinski, E. L., Hong, N. S., Tyndall, A. V., Halsall, B., & McDonald, R. J. (2010). Prefrontal cortical contributions during discriminative fear conditioning, extinction, and spontaneous recovery in rats. Experimental Brain Research, 203(2), 285–297. https://doi.org/10.1007/s00221-010-2228-0
Zwosta, K., Ruge, H., Wolfensteller, U., Heekeren, H. R., Noonan, M. P., & Farrer, C. (2015). Neural mechanisms of goal-directed behavior: outcome-based response selection is associated with increased functional coupling of the angular gyrus. https://doi.org/10.3389/fnhum.2015.00180